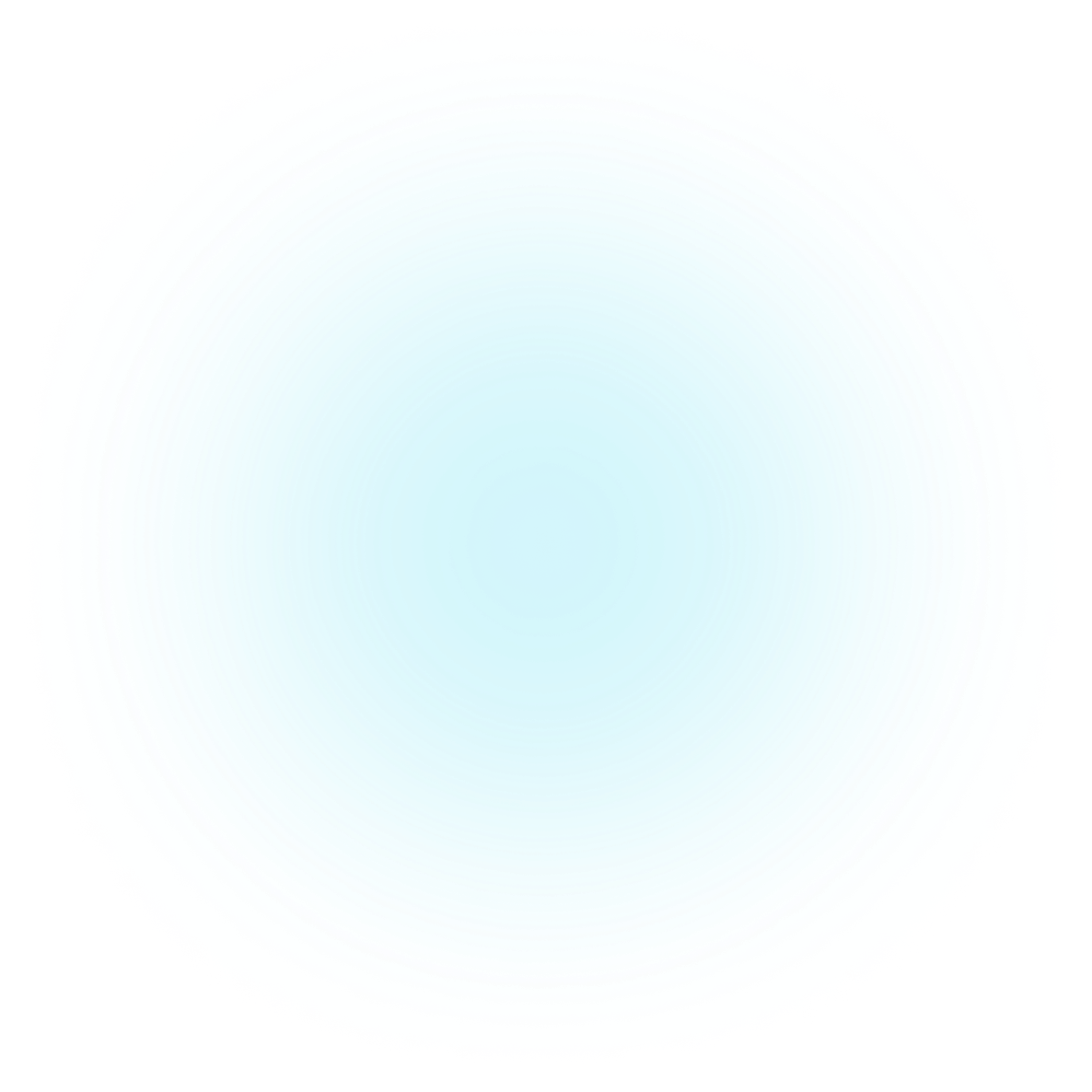
An open-source hub for all your molecular featurizers
Discover an unparalleled diversity of molecular featurizers and deploy them directly in your machine learning workflows.
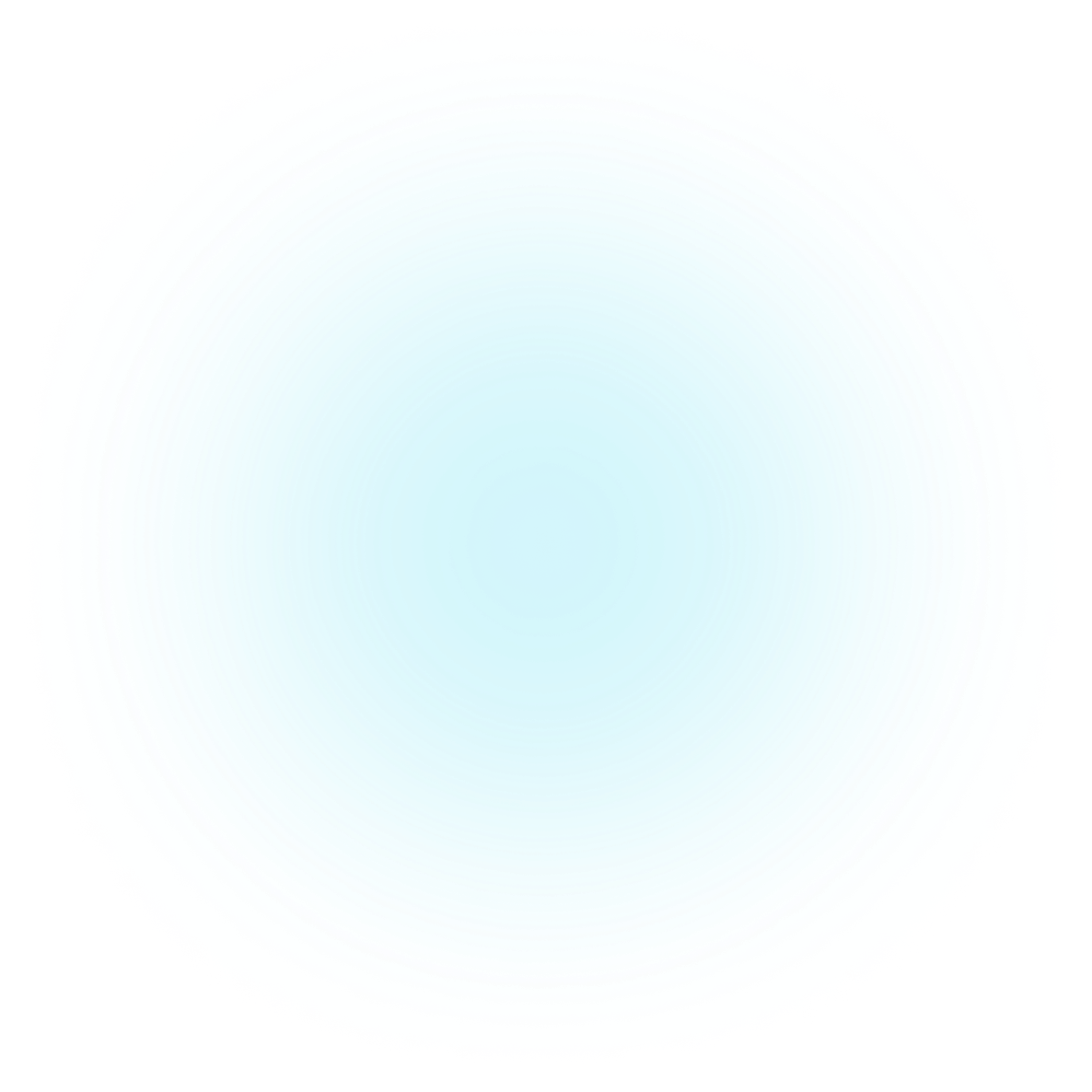
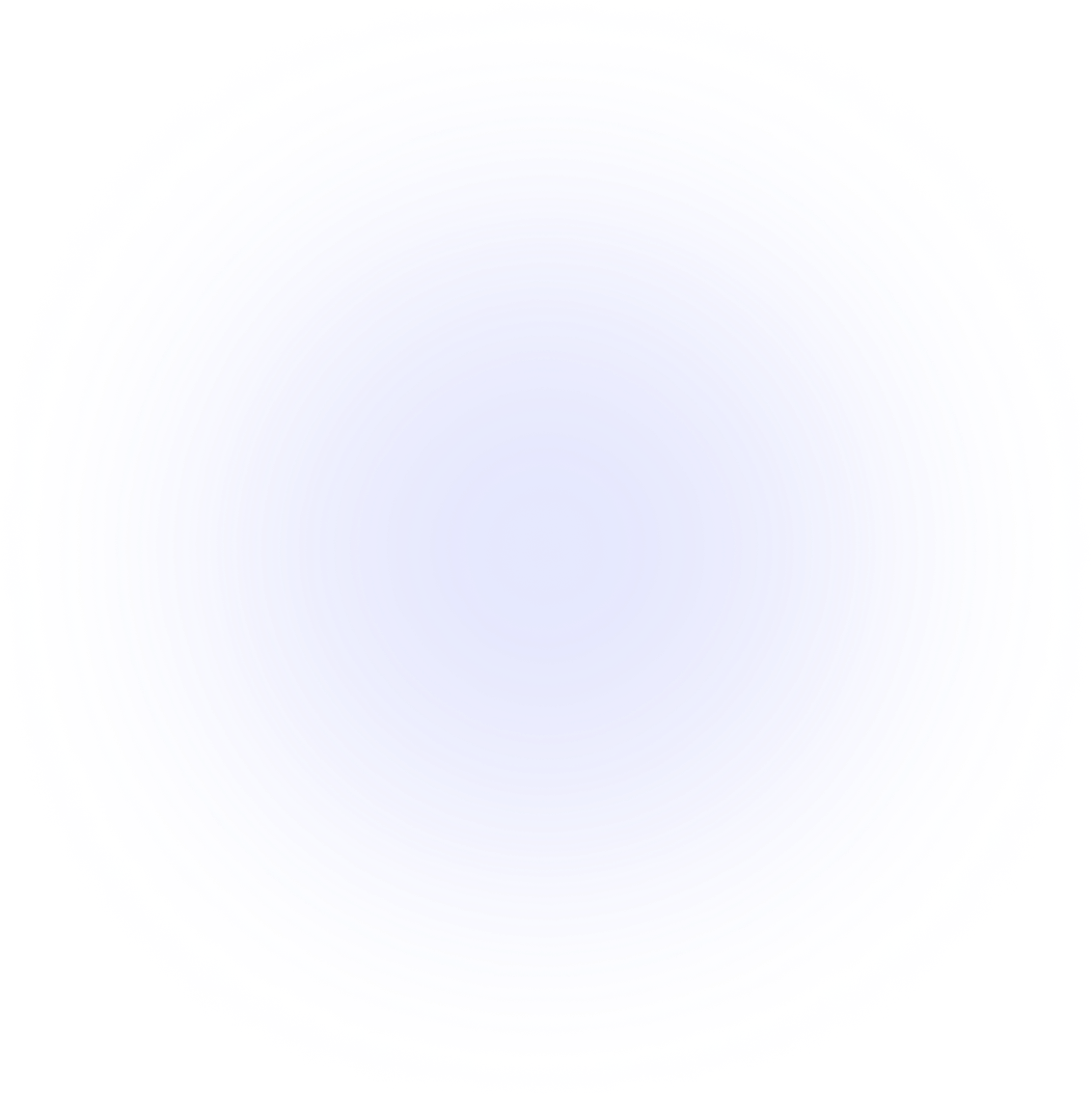
import datamol as dmfrom molfeat.calc import RDKitDescriptors2D
data = dm.data.freesolv().sample(500).smiles.valuesmol2d = data[83]
calc = RDKitDescriptors2D()calc(mol2d)
What is molfeat?
molfeat is an open-source hub that makes it easy for ML scientists to evaluate and implement a wide range of molecular featurizers. Find the right featurizer for your workflow today.
Roberta-Zinc480M-102M
This is a Roberta style masked language model trained on ~480m SMILES strings from the ZINC database. The model has ~102m parameters and was trained for 150000 iterations with a batch size of 4096 to a validation loss of ~0.122.
Updated on
GPT2-Zinc480M-87M
This is a GPT2 style autoregressive language model trained on ~480m SMILES strings from the ZINC database available. The model has ~87m parameters and was trained for 175000 iterations with a batch size of 3072 to a validation loss of ~.615.
Updated on
ChemGPT-1.2B
ChemGPT (1.2B params) is a transformer model for generative molecular modeling, which was pretrained on the PubChem10M dataset.
Updated on
ChemGPT-19M
ChemGPT (19M params) is a transformers model for generative molecular modeling, which was pretrained on the PubChem10M dataset.
Updated on
ecfp-count
The ECFP-Count (Extended Connectivity Fingerprints-Coun is essentially the same as the ECFP. However, instead of being hashed into a binary vector, there is no hashing process and simply a count vector is returned
Updated on
pcqm4mv2_graphormer_base
Pretrained Graph Transformer on PCQM4Mv2 Homo-Lumo energy gap prediction using 2D molecular graphs.
Updated on